Understanding Machine Learning Annotation Tools in Business
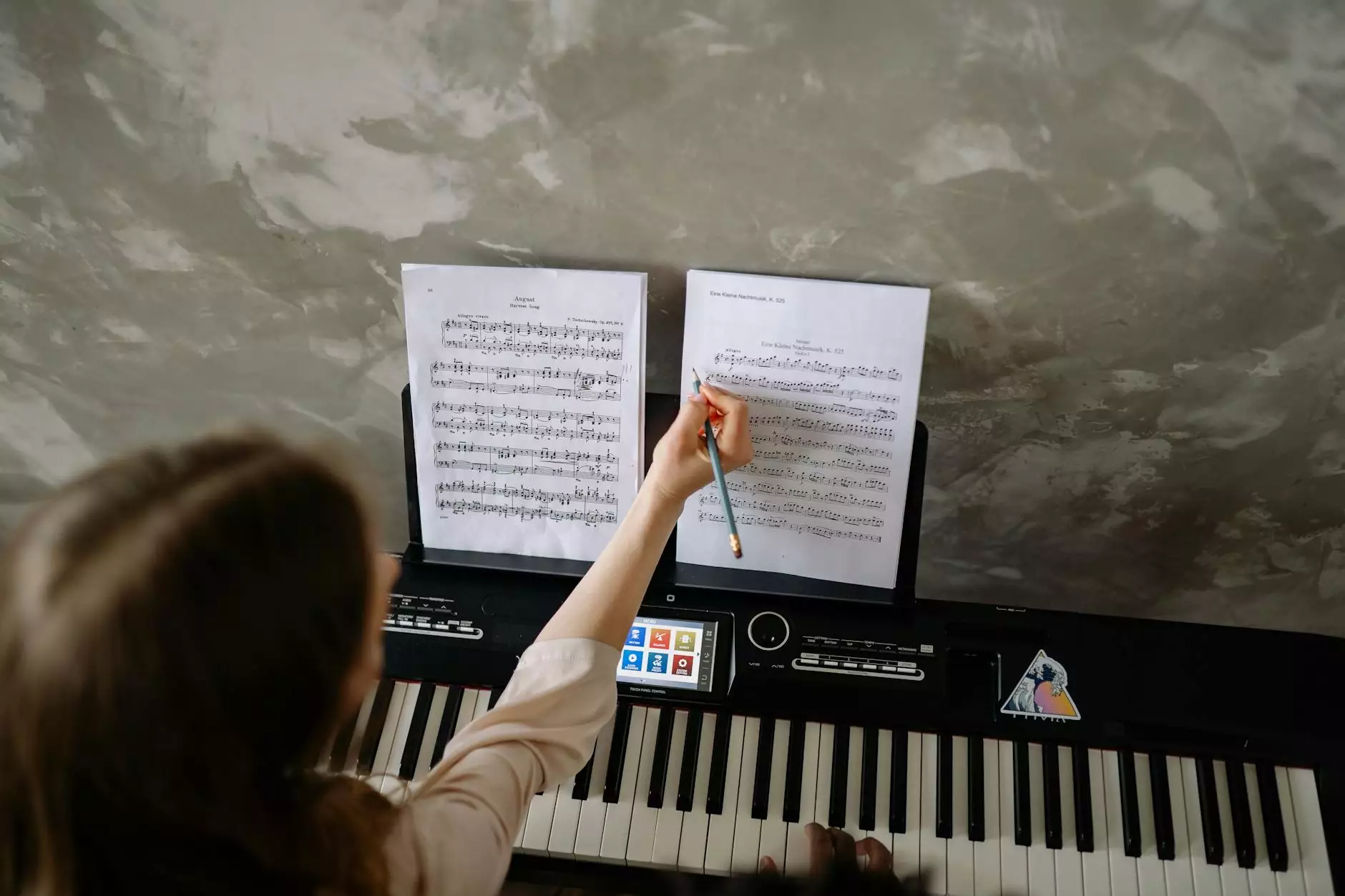
Machine learning annotation tools are becoming increasingly vital for businesses across various sectors, including home services, keys, and locksmiths. These tools facilitate the process of training machine learning models by providing the necessary data input in a structured format. In this comprehensive article, we will delve into the significance of machine learning annotation tools, explore their functionality, and highlight how they can revolutionize your business operations.
The Role of Machine Learning in Business
Machine learning stands at the forefront of the technological revolution, enabling businesses to leverage their data in unprecedented ways. In the context of the locksmith and home services industries, machine learning can optimize operational efficiency, enhance customer service, and drive profitability. Here's how:
- Predictive Analytics: By analyzing historical data, businesses can forecast trends and prepare for future demands.
- Enhanced Decision-Making: Machine learning algorithms can provide insights that empower better business decisions.
- Improved Customer Experience: Personalized services through machine learning can increase customer satisfaction and retention.
- Operational Efficiency: Automating routine tasks allows employees to focus on higher-level responsibilities.
What Are Machine Learning Annotation Tools?
Machine learning annotation tools are software applications designed to facilitate the process of labeling data. Annotation is crucial for supervised learning models, where the accuracy of outcomes depends heavily on the quality and quantity of labeled data. These tools simplify the annotation process, allowing businesses to prepare datasets effectively and efficiently.
Types of Data Annotations
Data can be annotated in various formats, depending on the requirements of the machine learning model:
- Text Annotation: Involves labeling pieces of text, such as entities, categories, or sentiments.
- Image Annotation: This includes drawing bounding boxes, polygons, or semantic segmentation on images.
- Audio Annotation: Labels sounds or voices for tasks like speech recognition or sentiment analysis.
- Video Annotation: Annotating video frames to identify objects, actions, or events.
Why Use Machine Learning Annotation Tools?
The advantages of using machine learning annotation tools are manifold. For businesses in the home services and locksmith industries, they can lead to significant improvements in various areas:
1. Improved Data Quality
High-quality, accurately annotated data is the backbone of effective machine learning models. These tools ensure that the data labeled meets stringent quality standards, thus leading to higher-performing AI applications.
2. Time and Cost Efficiency
Manual data labeling can be tedious and time-consuming, often leading to a bottleneck in the model training process. Machine learning annotation tools automate much of this work, saving valuable time and reducing costs. Automation not only speeds up the process but also minimizes human error, ensuring consistency across the dataset.
3. Scalability
As businesses grow, so does the volume of data they handle. Machine learning annotation tools can easily scale to manage larger datasets, making them an indispensable asset for growing organizations.
4. Enhanced Collaboration
These tools often feature collaborative functionalities, enabling multiple team members to work on data labeling simultaneously. This fosters teamwork and enhances the learning experience within the organization.
Implementing Machine Learning Annotation Tools in Your Business
To harness the power of machine learning annotation tools effectively, businesses should consider several strategies:
1. Identify Use Cases
Evaluate areas within your operations that could benefit from machine learning. Whether it’s enhancing customer service through chatbots or optimizing inventory management, pinpointing specific use cases is crucial.
2. Select the Right Tool
There are numerous machine learning annotation tools available, each with its strengths. Research and choose a tool that aligns with your business needs and the type of data you handle. Consider factors like:
- User-friendliness
- Integration capabilities with existing systems
- Support for custom annotation requirements
- Pricing models
3. Train Your Team
Invest in training your team on how to use these annotation tools effectively. Understanding the tool’s features and best practices will ensure you maximize its potential.
4. Monitor and Evaluate
Once implemented, continuously monitor the performance of your machine learning models. Regular evaluation helps identify areas for improvement in both the model and the data annotation processes.
Case Studies: Machine Learning in Home Services and Locksmiths
The application of machine learning in home services and locksmith industries is not just theoretical; several companies have successfully integrated these technologies to enhance their operations:
Case Study: KeyMakr.com
KeyMakr is an exemplary illustration of a modern locksmith firm that has embraced machine learning annotation tools to improve service delivery. By utilizing these tools, KeyMakr has been able to:
- Predict Customer Needs: By analyzing past service requests, KeyMakr can better anticipate customer requirements and allocate resources efficiently.
- Automate Customer Queries: Machine learning-driven chatbots handle customer inquiries, providing instant responses and freeing customer service representatives for more complex issues.
- Enhance Security Solutions: Leveraging annotated data helps KeyMakr develop cutting-edge security solutions tailored to customer specifications.
Case Study: Home Service Providers
Many home service providers have also integrated machine learning solutions to enhance their operational capabilities. These providers use data annotation to:
- Optimize Scheduling: Algorithms analyze historical data to optimize job scheduling based on location and technician availability.
- Improve Marketing Campaigns: Annotated customer data helps tailor marketing campaigns based on user preferences and behavior.
- Enhance Service Quality: Feedback loop systems utilize annotated data to continuously improve service strategies based on customer feedback.
Challenges in Implementing Machine Learning Annotation Tools
While the benefits are substantial, businesses may face challenges during the implementation of machine learning annotation tools:
1. Data Privacy Concerns
As data collection increases, so do concerns over privacy. Ensuring compliance with regulations such as GDPR is essential.
2. Quality Control
Maintaining data quality amidst higher volume can be challenging. Regular audits and updates are necessary to mitigate this risk.
3. Skill Gap
Finding employees with the requisite skills to effectively utilize machine learning tools can be difficult. Investing in training and support is crucial for success.
The Future of Machine Learning Annotation Tools in Business
The future of machine learning annotation tools looks promising, with rapid advancements in technology. We can expect:
- Increased Automation: Enhanced automation features will streamline the annotation process further.
- AI-Assisted Annotation: Tools will increasingly integrate AI to assist humans in the annotation process, minimizing time spent on repetitive tasks.
- Enhanced Collaborative Features: As remote work becomes more common, collaborative tools will play a crucial role in helping teams work together effectively.
Conclusion
Adopting machine learning annotation tools can provide significant advantages for businesses, especially in the home services and locksmith sectors. By improving data quality, enhancing operational efficiency, and fostering better customer experiences, these tools are vital for staying competitive in today’s fast-paced market. As machine learning technology continues to evolve, companies that embrace these tools will undoubtedly lead the way in shaping the future of their industries.