Unlocking the Potential: Agriculture Datasets for Machine Learning
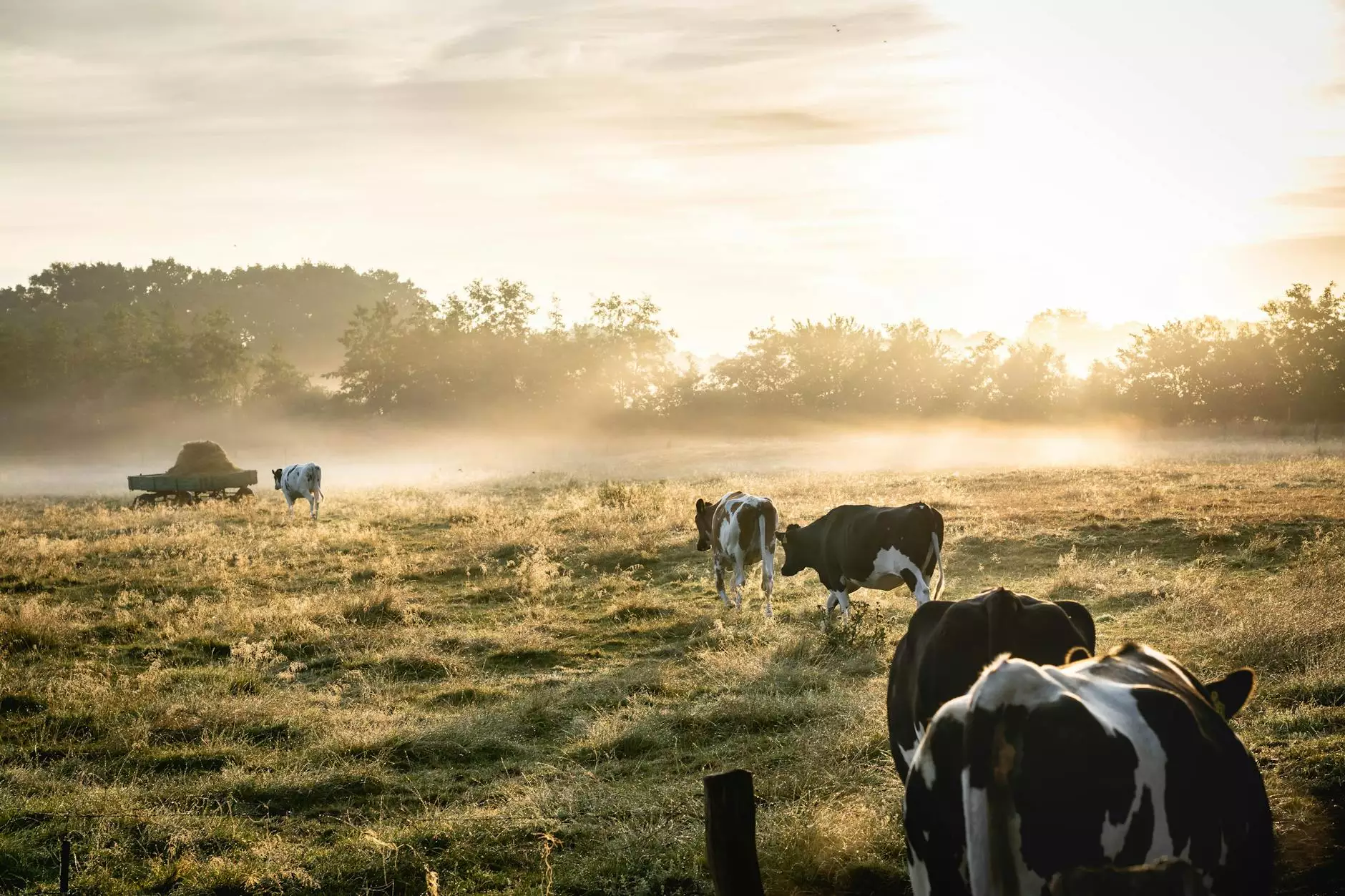
In the fast-evolving landscape of technology, the intersection between agriculture and machine learning is creating a paradigm shift. With the advent of advanced computational techniques and data analysis, farmers and agricultural scientists are leveraging agriculture datasets for machine learning to optimize farming practices, enhance yield, and ensure sustainability.
Understanding Agriculture Datasets
Agriculture datasets consist of a wide range of information collected from various agricultural activities. These datasets can include:
- Crop yield data - Information on the quantity of crops produced in different regions.
- Weather data - Historical and real-time weather patterns affecting agricultural output.
- Soil health indicators - Data on soil composition, pH levels, and nutrient content.
- Pest and disease incidence - Information on the occurrence of pests and diseases affecting crops.
- Market price trends - Data on the fluctuations in rates for various agricultural products.
By harnessing the power of these datasets, researchers and farmers alike can apply machine learning algorithms to analyze patterns, predict outcomes, and make informed decisions.
The Crucial Role of Machine Learning in Agriculture
Machine learning refers to the branch of artificial intelligence that focuses on the development of computer programs that can access data and use it to learn for themselves. In agriculture, machine learning models can help solve critical issues such as:
- Increased yield: By analyzing data from multiple sources, machine learning can help predict optimal crop conditions, resulting in higher productivity.
- Resource management: Machine learning helps in optimizing the use of water, fertilizers, and pesticides, promoting sustainable practices.
- Pest and disease prediction: Advanced algorithms can predict pest outbreaks and diseases based on historical data, reducing crop loss.
- Market prediction: Machine learning can analyze market trends, helping farmers make decisions about planting and selling their crops.
Benefits of Using Agriculture Datasets for Machine Learning
The application of agriculture datasets for machine learning offers numerous benefits:
- Enhanced Decision Making: With access to comprehensive data, farmers can make informed decisions that lead to better crop management.
- Predictive Analytics: Machine learning can forecast weather patterns and pest infestations, allowing proactive measures to be taken.
- Cost Reduction: By optimizing resource usage, farmers can significantly reduce operational costs.
- Improved Crop Quality: Analyses can lead to better crop management strategies that enhance the quality of produce.
- Sustainability: More efficient farming practices contribute to environmental sustainability by reducing carbon footprints.
Types of Agriculture Datasets for Machine Learning
When considering the use of agriculture datasets for machine learning, understanding the different types of datasets is crucial:
1. Remote Sensing Data
Remote sensing data, often obtained from satellites and drones, provides information on large agricultural areas. This data can be used to monitor crop health, moisture levels, and even soil composition.
2. Climate Data
Weather conditions have a significant impact on agriculture. Datasets that include temperature, rainfall, and humidity can help in predicting agricultural outcomes.
3. Soil Data
Details about soil types, nutrients, and geography are critical for understanding which crops are best suited to specific land features.
4. Yield and Production Data
Historical yield data can help researchers and farmers identify trends and make predictions about future production.
5. Socio-economic Data
Understanding the economic factors affecting agriculture, including market prices and labor availability, can greatly impact decision-making.
Challenges in Utilizing Agriculture Datasets
Despite the clear advantages, there are several challenges associated with the use of agriculture datasets for machine learning:
- Data Quality: Ensuring that data collected is accurate and reliable is essential for successful machine learning outcomes.
- Data Integration: Combining datasets from various sources can be complex and may require significant effort to standardize.
- Access to Technology: Not all farmers have access to the technology needed to implement machine learning solutions.
- Knowledge Gaps: A lack of understanding of machine learning can hinder effective utilization of the technology.
Case Studies in Agriculture Machine Learning
Various case studies illustrate the transformative potential of integrating agriculture datasets for machine learning in real-world farming scenarios:
1. Precision Agriculture with Drones
Farmers are using drones equipped with sensors to collect data on crop health. This information is fed into machine learning models that can provide insights on where to apply fertilizers or pesticides most effectively.
2. Smart Irrigation Systems
Machine learning algorithms analyze data from moisture sensors to optimize water usage on farms. This technology not only conserves water but also ensures crops receive exactly what they need to thrive.
3. Pest Management Solutions
Companies are developing machine learning models that predict pest infestations based on climate data and historical patterns, allowing farmers to act before an outbreak occurs.
Future of Agriculture Datasets and Machine Learning
The future of agriculture hinges on the successful integration of technology and data. Here are some trends to watch:
- Increased Automation: Automation in data collection will streamline processes and enhance data availability for machine learning applications.
- Better Data Sharing: Enhanced platforms for sharing agricultural datasets among farmers and researchers will promote collaboration and innovation.
- AI Advancements: Continuous advancements in artificial intelligence will lead to more sophisticated predictive models that can analyze complex agricultural systems.
- Focus on Sustainability: Emerging technologies will prioritize sustainability, helping farmers adopt practices that are economically viable and environmentally friendly.
Conclusion: Bridging the Gap Between Agriculture and Technology
The combination of agriculture datasets for machine learning represents a significant advancement in the agricultural sector. By analyzing vast amounts of data, farmers can make informed decisions that enhance productivity, promote sustainability, and mitigate risks. As technology continues to evolve, the agriculture industry will benefit from innovative solutions that address the challenges faced by farmers globally. The integration of machine learning into agricultural practices is not just a trend; it's a necessary evolution towards a more efficient, sustainable, and productive future.
agriculture dataset for machine learning